Penny-Wise, Pound-Foolish: The Oldest Warning for the Newest Technologies
- Severin Sorensen
- May 7
- 5 min read
"The sting of poor quality lingers long after the sweetness of low price is forgotten." – Attributed to John Ruskin, Benjamin Franklin, and generations of business leaders
Across centuries of commerce, one strategic tension remains constant: the trade-off between low cost and high quality. While low prices may win in the short term, history shows us that compromised quality often leads to long-term failure—whether in reputational damage, financial loss, or operational collapse.
In today’s AI-powered economy, this lesson is more urgent than ever. As organizations scale faster and automate more decisions, the temptation to reduce costs—be it through leaner data sets, accelerated deployments, or cheaper talent—is omnipresent.
But as Benjamin Franklin warned centuries ago, being penny-wise and pound-foolish may deliver quick wins while sowing the seeds of strategic regret.
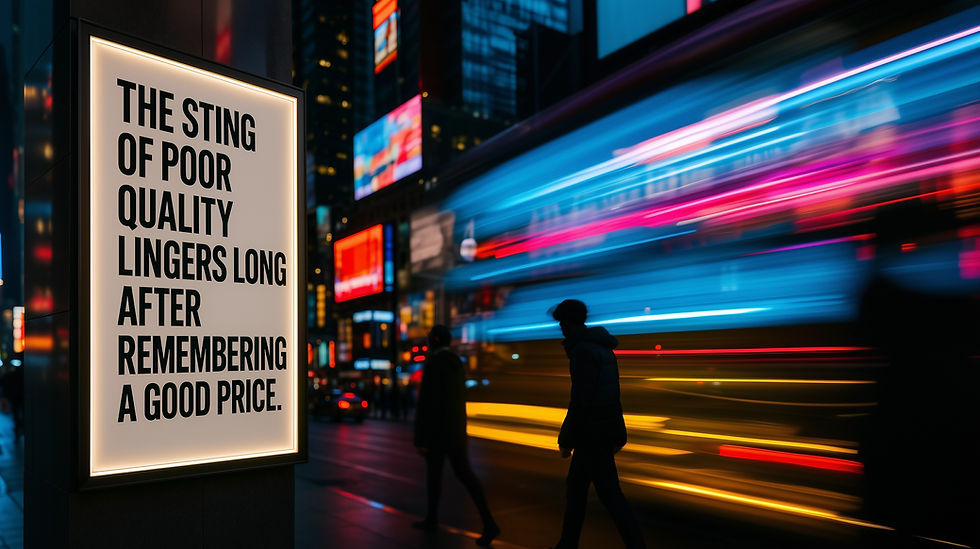
This article was originally published on LinkedIn by Severin Sorensen and has been approved for placement on Arete Coach. Scroll to continue reading or click here to read the original article.
The Strategic Cost of Poor Quality: From Ford Pinto to Algorithmic Drift
Throughout modern business history, we’ve seen how short-term cost-cutting can lead to catastrophic outcomes:
Ford Pinto (1970s): A known safety flaw cost $11 per unit to fix—but was ignored. The result: dozens of deaths, lawsuits, a massive recall, and irreparable reputational damage.
BP Deepwater Horizon (2010): Cost-saving decisions on safety and materials triggered one of the worst environmental disasters in history.
Volkswagen Dieselgate (2015): Software designed to cheat emissions tests saved costs—until it triggered over $30 billion in penalties and a global loss of trust.
These failures were not operational flukes. They were cultural decisions, made when leadership valued short-term cost savings over systemic quality and integrity.
From Deming to Drucker: Quality Is a Management System
W. Edwards Deming didn’t see quality as a department or inspection step. He saw it as a system-wide responsibility, requiring leadership commitment, supplier alignment, and continuous improvement. His 14 Points for Management were clear:
“End the practice of awarding business on the basis of price tag alone.”
“Improve constantly and forever the system of production and service.”
Peter Drucker added the customer lens. In his view, quality is not what the company puts in—it is what the customer gets out and is willing to pay for. This means quality must be measured in outcomes, not intentions.
Together, Deming and Drucker offer a blueprint for leaders: align quality with purpose, value, and trust—not merely with cost control.
Artificial Intelligence: A New Arena, Same Old Mistake
AI introduces unprecedented scale and efficiency—but also new risks when quality is neglected:
Low-quality training data introduces bias, reduces accuracy, and undermines fairness.
Accelerated deployment cycles often skip testing, model validation, or ethical review.
Optimizing for cost-per-output, without alignment to broader business or societal values, leads to algorithmic drift and decision-making opacity.
In short: AI amplifies the values of the systems it's embedded in. If your organization is focused only on cost minimization, your AI will replicate that bias—at scale.
The Hidden COPQ of AI: Cost of Poor Quality in the Algorithmic Age
Traditionally, the Cost of Poor Quality (COPQ) includes rework, recalls, warranty claims, and loss of customer trust. In AI, these costs take new forms:
Classical COPQAI-Age EquivalentProduct reworkModel retraining and bias mitigation
Warranty claimsCompliance fines or consumer lawsuitsBrand reputation damagePublic backlash or media exposésLost customer loyaltyAlgorithmic exclusion or unfair targeting
The most dangerous costs are often invisible—until they become existential.
Leadership Imperative: Don't Let the Algorithm Outpace Your Judgment
Leaders must reject the false dichotomy between innovation and integrity. Quality must be designed into AI systems from the start:
Build strong foundations: robust data governance, training protocols, ethical review boards.
Align incentives: reward teams not just for speed, but for resilience, explainability, and fairness.
Embed human oversight: critical decisions need judgment, not just optimization.
“There is nothing so useless as doing efficiently that which should not be done at all.” – Peter Drucker
Speed without purpose. Scale without ethics. Automation without quality. These are the real risks.
A Modern Reading of Franklin: Penny-Wise and Pound-Foolish in the AI Era
Franklin’s timeless aphorism applies with new force:
Penny-wise: Choosing cheaper datasets, faster deployment, or less-experienced vendors.
Pound-foolish: Facing downstream costs of rework, litigation, brand erosion, and algorithmic harm.
Just as cutting corners in manufacturing led to the downfall of companies like Schlitz Beer or J.C. Penney, cutting corners in AI can erode public trust, investor confidence, and stakeholder value.
The ROI of quality—whether in process, product, or AI system—is not theoretical. It’s historical. It's measurable. And it's increasingly non-negotiable.
The Silent AI Surge: What CEOs Are Missing—and Risking
Recent workforce surveys reveal a striking and urgent reality: the number of employees using AI tools for work exceeds the number of companies that have formally authorized or provisioned AI accounts. This quiet revolution unfolding across industries holds two serious implications for business leaders:
Unintentional Data Leakage at Scale
When employees use consumer-grade AI tools without corporate guidance or governance, proprietary data—including client details, internal strategies, financials, and product plans—can inadvertently be exposed to third-party platforms. In many cases, these platforms are not subject to company-level controls, compliance standards, or data retention policies.
This creates a shadow AI risk surface, where sensitive information is shared outside secure systems—without malicious intent, but with potentially catastrophic consequences.
Quality and confidentiality are inseparable. When governance is absent, risk is present—by default.
Underutilized Talent and Untapped Strategic Advantage
At the same time, organizations failing to provide secure, sanctioned AI tools are missing the opportunity to harness the collective intelligence and productivity of their workforce. Employees are clearly signaling a willingness—even eagerness—to use AI to improve output. But without access to company-aligned tools, frameworks, or training, much of this potential is squandered or misdirected.
Rather than resisting AI adoption, CEOs should focus on channeling it safely, strategically, and systemically.
The CEO’s Mandate: Govern, Guide, and Leverage AI
This trend demands more than an IT response—it calls for executive vision and operational leadership:
Implement clear AI usage policies rooted in data security, transparency, and ethical use.
Provision enterprise-grade AI tools that protect IP and reflect company values.
Train teams to use AI thoughtfully—not to replace critical thinking, but to augment it.
Embed quality gates into AI usage, just as you would with any mission-critical system.
The quality of your AI adoption will reflect the quality of your leadership.
Conclusion: The Standard for Leadership Is Rising
In the AI era, the most important competitive advantage is trust. And trust is built on quality—of experience, of ethics, and of outcomes.
The best leaders won’t just chase efficiency. They will build legacies of value, grounded in systems that prioritize what matters most.
Because in the end, your customers—and your algorithms—will remember how you built, not just what you built.
Copyright © 2025 by Arete Coach LLC. All rights reserved.
Comentarios